MNIST数据集是手写数字0~9的数据集,一般被用作机器学习领域的测试。
本程序先导入数据,再利用最小梯度法进行训练使得样本交叉熵最小,最后给出训练之后程序的准确率。
交叉熵的定义:
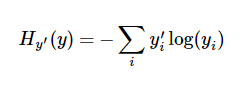
y 是我们预测的概率分布, y’ 是实际的分布。
该指标用来衡量学习结果与实际情况的差距。
import tensorflow.examples.tutorials.mnist.input_data as input_data import tensorflow as tf # initialize mnist = input_data.read_data_sets("F:\Tensorflow\MNIST", one_hot=True) x = tf.placeholder(tf.float32, [None, 784]) W = tf.Variable(tf.zeros([784, 10])) b = tf.Variable(tf.zeros([10])) y = tf.nn.softmax(tf.matmul(x, W) + b) y_ = tf.placeholder("float", [None, 10]) cross_entropy = -tf.reduce_sum(y_ * tf.log(y)) train_step = tf.train.GradientDescentOptimizer(0.01).minimize(cross_entropy) # train init = tf.initialize_all_variables() sess = tf.Session() sess.run(init) for i in range(1000): batch_xs, batch_ys = mnist.train.next_batch(100) sess.run(train_step, feed_dict={x: batch_xs, y_: batch_ys}) # predict correct_prediction = tf.equal(tf.argmax(y, 1), tf.argmax(y_, 1)) accuracy = tf.reduce_mean(tf.cast(correct_prediction, "float")) print(sess.run(accuracy, feed_dict={x: mnist.test.images, y_: mnist.test.labels}))
先用placeholder和variable初始化。placeholder(占位符)一般用于导入数据,而Variable一般是与学习过程相关的变量。
之后,启动Session进行训练,本程序当中随机选取了100张图片进行训练。
最后运行的准确率为91.63%。如下图:
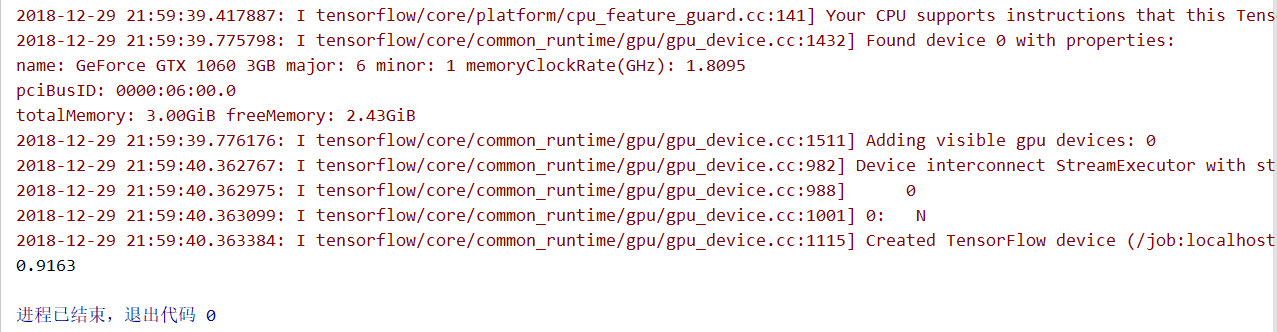